Mastering AI in Data Analytics: Comprehensive Guide to AI Tools for Data Analytics and Next-Generation Techniques
Welcome to the fascinating world of AI in data analytics! Gone are the days of tediously crunching numbers and manually sorting through data. AI has revolutionized the way we handle, analyze, and interpret data, making it more efficient, accurate, and insightful. But how exactly does this work? Let’s dive in!
What is AI in Data Analytics?
AI in data analytics refers to the use of machine learning algorithms and other AI techniques to analyze and interpret complex data sets. This isn’t just about processing data faster; it’s about uncovering patterns, predicting trends, and making sense of data in ways that were previously impossible or extremely time-consuming.
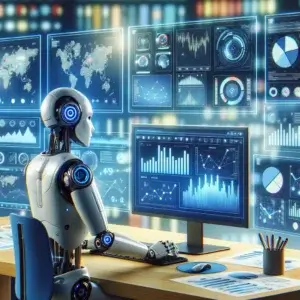
How is AI used for data analysis?
AI, the emulation of human intelligence by machines, stands in contrast to data analytics, which entails scrutinizing raw data to unveil trends and address queries. Together, AI amplifies the potential of data analytics, ushering in more refined and predictive insights crucial for data-driven decision-making.
Key Components of AI in Data Analytics
- Machine Learning: AI systems learn from data, identifying patterns and making predictions.
- Natural Language Processing (NLP): AI understands and interprets human language, enabling it to extract insights from text data.
- Predictive Analytics: AI predicts future trends based on historical data.
- Data Mining: AI discovers patterns and relationships in large data sets.
Benefits of AI in Data Analytics
- Efficiency: AI can process and analyze data much faster than a human can.
- Accuracy: AI reduces the risk of human error, leading to more accurate data analysis.
- Insightful Predictions: AI can predict future trends, helping businesses to make informed decisions.
- Automation: Routine data analysis tasks can be automated, freeing up human analysts for more complex tasks.
Real-World Applications
- Healthcare: AI analyzes patient data to predict health risks and outcomes.
- Finance: AI is used for risk assessment, fraud detection, and algorithmic trading.
- Retail: AI predicts consumer behavior and trends, optimizing inventory and marketing strategies.
- Transportation: AI analyzes traffic data to improve route planning and reduce congestion.
The Human Touch in AI-Driven Data Analytics
While AI brings numerous advantages, the human element remains crucial. AI systems are designed and programmed by humans, and human oversight is necessary to ensure ethical and responsible use of AI in data analytics. Interpretation of data often requires human insight, especially when it involves complex social or cultural factors.
Challenges and Considerations
- Data Privacy: The use of AI in data analytics raises concerns about data security and privacy.
- Bias in AI: AI systems can inherit biases from the data they are trained on, leading to skewed results.
- Complexity: Implementing AI in data analytics can be complex and require significant investment.
The Future of AI in Data Analytics
The future of AI in data analytics is promising, with continual advancements in technology leading to more sophisticated and intuitive AI tools. As AI becomes more integrated into various sectors, its role in data analytics is set to become more pivotal, driving innovation and efficiency across industries.
AI vs. Data Analytics: Understanding the Differences and Synergies
AI refers to the simulation of human intelligence in machines, while data analytics involves examining raw data to find trends and answer questions. When combined, AI amplifies the capabilities of data analytics, enabling more nuanced and predictive insights, which are crucial for data-driven decision-making.
Unveiling AI Techniques in Big Data Analytics
Big data analytics is significantly enriched by AI techniques such as deep learning, which can analyze vast and complex datasets, and natural language processing (NLP), which interprets and understands human language in a meaningful way. These techniques are crucial in handling the scale and complexity of big data.
AI’s Role in Business Analytics
AI in business analytics has become a cornerstone for deriving strategic insights. AI tools help in forecasting market trends, understanding customer behavior, and automating routine tasks, thereby enhancing efficiency and accuracy in business decisions.
The Future of Data Analysis: Will AI Replace Data Analysts?
While AI can automate numerous analytical tasks, it is not poised to replace data analysts. Instead, AI acts as a powerful tool to augment human capabilities, allowing analysts to focus on more strategic and interpretive aspects of data analytics.
The Intersection of Big Data Analytics and AI
AI and big data analytics are intrinsically linked. AI’s ability to process and analyze vast amounts of data quickly and accurately makes it an essential element of big data analytics strategies.
A Comprehensive Guide to AI tools in Data Analytics:
What is an AI Data Analytics Tool?
An AI Data Analytics Tool is a sophisticated software application that uses machine learning, data mining, and predictive analytics to process and analyze data. Unlike traditional data analytics, these tools can handle vast quantities of data with greater accuracy and efficiency, often uncovering patterns and insights that would be impossible for humans to detect manually.
Types of AI Tools for Data Analytics
Descriptive Analytics Tools (What Happened): Tools like Tableau and Microsoft Power BI help visualize data trends and patterns.
Diagnostic Analytics Tools (Why It Happened): Tools like Qlik and Google Analytics provide insights into data correlations and causations.
Predictive Analytics Tools (What Could Happen): SAS Analytics and IBM SPSS offer advanced modeling capabilities for forecasting future trends.
Prescriptive Analytics Tools (What Should Be Done): Alteryx and RapidMiner guide decision-making by suggesting actions based on data predictions.
Best AI Data Analytics Tools
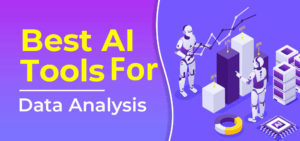
Tool Name | Description of the Tool |
---|---|
TensorFlow | An open-source software library developed by Google for numerical computation and machine learning. TensorFlow’s flexible architecture allows for easy deployment across various platforms. TensorFlow’s strengths lie in its versatility and scalability, enabling its deployment across various platforms, from individual computers to large servers, and even mobile devices. It’s particularly favored for tasks that require deep learning, as it simplifies the creation and training of machine learning models. The tool’s intuitive design and comprehensive library of functions make it accessible not just to experts but also to newcomers in the field of data analytics and AI. |
Apache Spark | A unified analytics engine for large-scale data processing, known for its speed, ease of use, and sophisticated analytics capabilities. Apache Spark is a powerful open-source data analytics tool known for its speed and ease of use in handling big data tasks. It’s a versatile platform designed to efficiently process large-scale data across a distributed computing environment. Spark excels in real-time data processing, making it ideal for applications requiring quick insights from massive data sets. Its ability to handle batch processing, streaming data, machine learning, and interactive queries all in one framework sets it apart. Developers appreciate Spark for its simple programming interface, which supports multiple languages like Scala, Python, and Java, allowing for easy integration with existing systems. Spark’s robust community and continuous development ensure it remains a top choice for businesses and researchers dealing with extensive and complex data analysis. |
IBM Watson | IBM Watson Analytics comes with a cool feature that lets you chat with your data like you would in a normal conversation, thanks to its natural language processing abilities. This makes digging through and analyzing data feel more natural and easy. It automatically spots trends and important patterns for you, helping you see connections in your data that you might have missed. Plus, its predictive modeling means you can make smart, data-backed choices by using the smarts of AI algorithms. It offers advanced AI capabilities, including natural language processing and machine learning, tailored for business needs. |
H2O.ai | An open-source AI platform that simplifies the creation of smart applications using machine learning algorithms. H2O.ai makes machine learning easy for everyone, not just the experts. With its automated tools, you can quickly create and use models without needing to be a data science pro. It uses smart algorithms, like gradient boosting and deep learning, to make really accurate predictions. Plus, it’s designed to handle big data smoothly and efficiently, so you can work with lots of information without a hitch. |
DataRobot | A machine learning platform that enables data scientists of all skill levels to build and deploy accurate predictive models quickly. DataRobot’s tool is like having a super-smart assistant for machine learning. It takes care of the heavy lifting in building models by automating the process, which means you don’t have to do everything by hand. Imagine it as a smart chef in a kitchen who knows exactly which ingredients (algorithms and parameters) will make the perfect dish (model). This means you get top-notch models that work really well, without spending ages figuring out the nuts and bolts. Plus, the AI algorithms in this tool are really sharp, helping you make smart choices based on what the data is telling you. |
SAS Analytics | |
Tableau | A powerful data visualization tool that transforms raw data into easily understandable visual formats, enhancing data analysis and decision-making. Tableau makes looking at data easy and fun with its user-friendly dashboards. You can play around with data and see it in different visual forms. Plus, its cool AI feature called ‘Ask Data’ lets you just ask questions like you would to a friend and get answers in neat visuals right away. It also uses smart machine learning to dig deeper into data, helping you get more meaningful insights. |
Microsoft Power BI | |
RapidMiner | An advanced analytics platform that offers an integrated environment for data preparation, machine learning, deep learning, text mining, and predictive analytics. RapidMiner makes data analysis easy for everyone, no matter their tech skills. It has a simple, drag-and-drop system that lets you build workflows effortlessly. This tool does the heavy lifting by automatically setting up your data and creating predictive models, which means you save a lot of time and hassle. Plus, it comes packed with a bunch of ready-to-use AI models, so you can use tried-and-tested methods for quick and efficient analysis. |
Alteryx | A tool for data science and analytics that offers data blending, advanced analytics, and data sharing capabilities to accelerate the data analytics process. Alteryx makes it a breeze to get your data ready for analysis. It cuts down the manual work usually needed to blend and prepare data. With its tools for predictive and spatial analytics, you can dive deeper into your data and make smarter decisions. Plus, Alteryx automates those tricky data workflows, boosting productivity. This means companies can handle big data projects more efficiently, making the most of their data with Alteryx. |
Each of these tools brings unique capabilities to the field of AI in data analytics, helping businesses and data professionals to extract meaningful insights from complex data sets.
ChatGPT and Data Science: Complement or Replacement?
ChatGPT and similar AI models are more complementary to the role of data scientists. They aid in initial data processing and analysis but cannot fully replace the advanced understanding and critical thinking skills of human experts.
Conclusion:
Additional Resource:
For more on AI’s technical aspects, from data processing to model deployment, visit How AI Works: From Data Processing to Model Deployment.